Data Quality: The Hidden Competitive Advantage
Podcast Player in here
In today’s digital-first insurance world, data has shifted from being a byproduct to a strategic powerhouse. In this candid discussion, Tony from COVER interviews Angus Black, co-founder of BarnOwl Data Solutions, about the transformation unfolding in the insurance space around data quality, management, and analytics.
Angus shares how data volumes have exploded due to digital transformation and evolving business demands—everyone wants to make decisions backed by solid data. But more data doesn’t always mean better data. He unpacks the reality of legacy systems, data that was never structured for modern use, and the high cost of going back to fix what was once “good enough.”
BarnOwl’s work revolves around helping insurers and brokers define what “quality data” means for their business, measure it through scoring mechanisms, and work with the source—usually brokers—to clean and enrich it. The conversation also touches on external data integration, from validating addresses and IDs to incorporating GPS coordinates and weather data to add meaningful context.
Angus also speaks about the ongoing nature of this work—it’s not a once-off cleanup, but an evolving process embedded into operations. The interview closes on skills shortages in the data space, and how roles focused specifically on data quality are beginning to emerge.
This episode is a must for anyone in the insurance value chain seeking to transform how they handle data—because data isn’t just a support function anymore, it’s a competitive differentiator.
Key Points
- Data explosion: Massive growth in data volumes driven by demand for data-driven decision-making.
- Quality shift: Quality expectations for data have increased—it's no longer just about having data, but having the right data.
- Legacy challenges: Old systems and evolving insurance products create inconsistent, difficult-to-use legacy data.
- Defining quality: Quality data is data that can answer business questions and inform decisions.
- Measuring quality: Using scoring systems and ongoing monitoring helps track and improve data health.
- Enrichment & validation: External databases (e.g., for ID, location, weather, crime) help verify and enhance internal data.
- Continuous process: Cleaning and maintaining data quality is an ongoing, operational necessity—not a once-off project.
- Skills gap: There’s a growing need for professionals focused specifically on data quality.
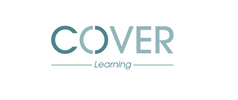
COVER Learning
Introductory to Advanced. Online Qualifications, Business, CPD, RE preparation. View some of our latest courses below.